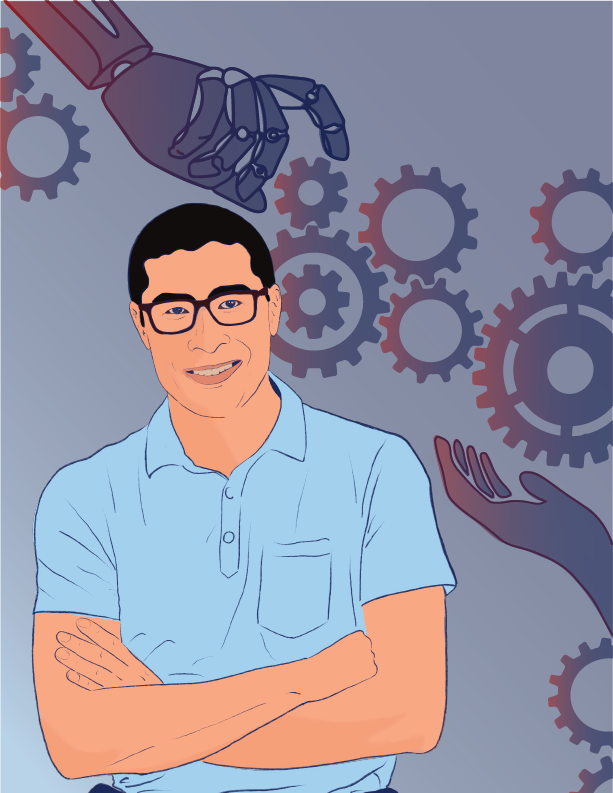
Read original Article
Robots are now helping people compose emails and vacuum their homes, but we are still far away from having a Jetson’s-style, live-in robot to help with more complex tasks.
“I want a robot to be able to enter a room it has never been in and quickly characterize and manipulate objects to perform an assigned task,” says Michael Posa, Assistant Professor with appointments in Mechanical Engineering and Applied Mechanics, Computer and Information Science, and Electrical and Systems Engineering. “Whether that’s assisting in the home, conducting search and rescue operations or manufacturing items.”
But a robot is only as smart as we train it to be. The reason we have not yet been able to create robots with this real-world intelligence is due to the way we are teaching them. Robots currently learn through repetitive training in simulations and controlled laboratory settings. They may be great at performing an extremely precise motion over and over again, but have a hard time quickly reacting to diverse stimuli in an uncontrolled environment. To improve that ability, we need to teach robots how to process information in novel environments where there is no time or opportunity to waste.
With funding from the National Science Foundation’s CAREER Award, Michael Posa is working on a new teaching method where robots interact with objects in the real world and observations from those interactions are used to create a training lesson plan or model. The new approach is real world first, simulation second, a prioritization which Posa believes is key to building robots’ real-world intelligence.
To accomplish this, we must enable robots to learn from small data sets. Unlike machine learning models like ChatGPT, where big data sets of language, images and video can be found on the internet, data in robotics is hard to come by. Advanced robots remain expensive and require specialized skills to operate. While shared data sets are growing in scale, they are many orders of magnitude smaller than what is available in big data sets.
“As an example, for a robot to prepare meals in the home, it must master a huge range of different foods, tools and cooking strategies,” says Posa. “While humans intuitively understand and learn from small data – teaching a human how to prepare a meal just once or twice would be enough for them to successfully accomplish the task on their own – computers require far more repetition in an environment with little to no interruption.”
In addition to working with small data, future life-improving robots will need to be able to perform and react to many different, notoriously hard-to-model, discontinuous movements such as jumping, sticking, sliding, chopping and bouncing, all while adhering to the laws of physics.
“Initial studies started with simulations, but we’re now focused on training robots using a model based on real-world observations,” says Posa. “We are currently working through the fundamentals of the physics and math required to create a successful model, and we have already started working with robotic arms and hands to test and improve dexterity with our new teaching approach.”
With research underway, Posa plans to create a cloud-based lesson plan accessible to students around the world, using the award to bridge gaps in both research and outreach.
“Our future engineers are learning algebra and calculus now,” says Posa. “We want to show them how what they are learning in school relates to a real-world problem, solution and career path in robotics.”