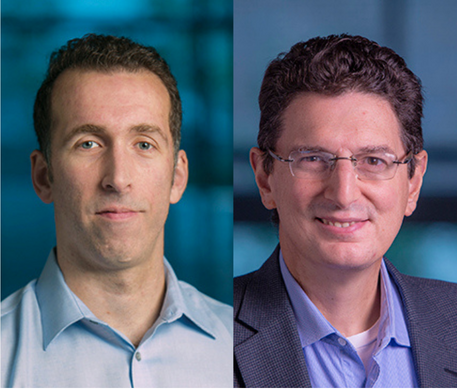
Text by Jillian Mallon
This article is one of a two-part series on the GRASP Lab’s involvement in the NSF’s new Safe Learning-Enabled Systems program. The article below covers the proposal in the foundation category that was developed by GRASP faculty members Dr. Nikolai Matni and Dr. George Pappas. Click here to read the article that covers the proposal that GRASP faculty member Dr. Dinesh Jayaraman contributed to in the synergy category.
Artificial intelligence-enabled platforms have become more widely available in a greater number of applications in the past year. Since the release of ChatGPT in November 2022, companies have launched their own AI platforms such as Google’s Bard and Adobe’s Firefly. Despite the growing accessibility, the reliability of these applications is still in question. Universities including the University of Pennsylvania have implemented guidelines for the safe use of predictive speech tools due to an increased risk of errors and plagiarism. This is just one reason the machine learning community has a need to develop ways to ensure the safety of AI platforms.
The National Science Foundation debuted its new Safe Learning-Enabled Systems (NSF SLES) program this year in search of ideas that would increase the safety of systems that are equipped with artificial intelligence. The competitive grant allocated its $10.9 million in funding in its inaugural year to only 11 projects across the nation in two different categories: foundation and synergy. Despite such a limited selection of winners, GRASP Laboratory-affiliated faculty members at Penn were awarded funding for one project in each category.
GRASP Professors Dr. Nikolai Matni and Dr. George Pappas collaborated on a foundational proposal titled “Bridging offline design and online adaptation in safe learning-enabled systems”. The proposal outlines a novel approach to the NSF SLES program’s goal of end-to-end safety. Dr. Matni and Dr. Pappas plan to ensure end-to-end safety by creating a feedback loop in which the two main phases of the system communicate their findings to each other and integrate this data into a safer version of the system.
The lead principal investigator of this proposal, Dr. Matni, explained this novelty by stating, “There have been lots of notions of what end-to-end safety could mean. Some people might call a guarantee on the full system rather than the individual components ‘end-to-end’. We actually took a slightly different tack in this project. Rather than considering end-to-end in the context of the system, we actually considered end-to-end in the context of designing and deploying a learning-enabled system.”
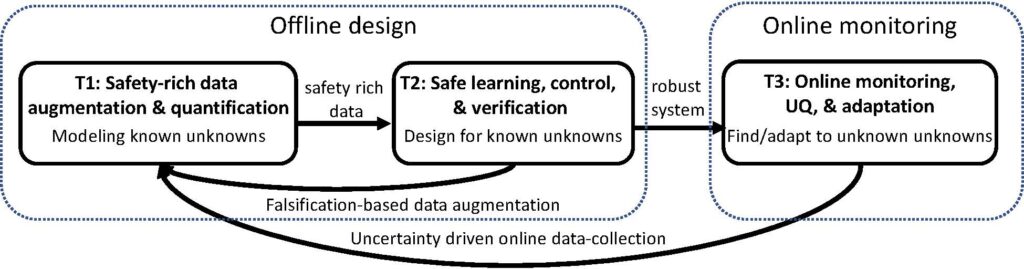
The first phase of the project is the offline design process of the system. In this phase, the researchers will program the system to recognize and respond to “known unknowns”, or problems that it might encounter that can be predicted before the system is deployed.
“This is where I do most of the machine learning,” explained Dr. Matni, “I try to do that in as holistic and as comprehensive a way as possible to try to account for any kinds of different scenarios that you might see in the real world. So you might do something like randomize the kinds of environments that your system is trained in, or even try to do adversarial training, which is, of all of the things you expect to see, trying to pick out the worst thing that you might see, and then make sure that your system is robust to that.”
This proposal identified two intellectual challenges within the offline design process. The first challenge is to model potential known unknowns through developing data augmentation methods that replicate real-world uncertainty and through identifying what safety-rich data should be collected. The second challenge is to incorporate these data augmentation methods into robust learning and control frameworks to determine the amount of data needed to train an AI-enabled system on safety.
“Another major novelty of this proposal is that we want to try to characterize what we call ‘safety rich data’, which is that in machine learning not all data is equal. Some data could be more useful to help us understand uncertainty and become more safe as opposed to just generic data,” noted Dr. Matni, “Suppose that I have a quadrupedal robot and most of the time it’s just walking on flat ground. All that data from walking on flat ground isn’t super informative. But then, suppose it steps on a slippery patch of water or oil and slips. I get to collect that data. That data should be much more informative in terms of how to avoid slipping the next time around. What is lacking right now is a quantitative theory to actually disambiguate in a very precise way what data is more important than others for the kind of context of safety, and so we’re planning on also evaluating that using robotic platforms.”
The second portion of this cycle is the online deployment phase during which the system is actually integrated into a robot or learning-enabled device and run in a real life scenario. The system will be trained to handle “unknown unknowns”, or problems that were not considered or predicted in the offline design process. This would involve arming the system with tools that can characterize and monitor possible issues with the system and adapt the system components in real time. The system will also collect data on previously “unknown unknowns” during the online deployment phase so that the new findings can be fed back into the offline design process.
“In the online deployment phase, you encounter things that you weren’t expecting. One of our ideas is to try to recognize when you’re in that situation and seeing things that are completely outside of the scope of what you’ve been trained on, and then balance between being safe and trying to get more information about this unknown unknown so that it could be incorporated into the next iteration of the offline design phase,” described Dr. Matni, “I’m okay with losing a few robots, but eventually I want to make sure that my robot is going to be successful within an environment. If we do this over and over again, eventually we’ll have collected all of the data needed to characterize even the unknown unknowns. Our notion of end-to-end safety is closing this gap.”
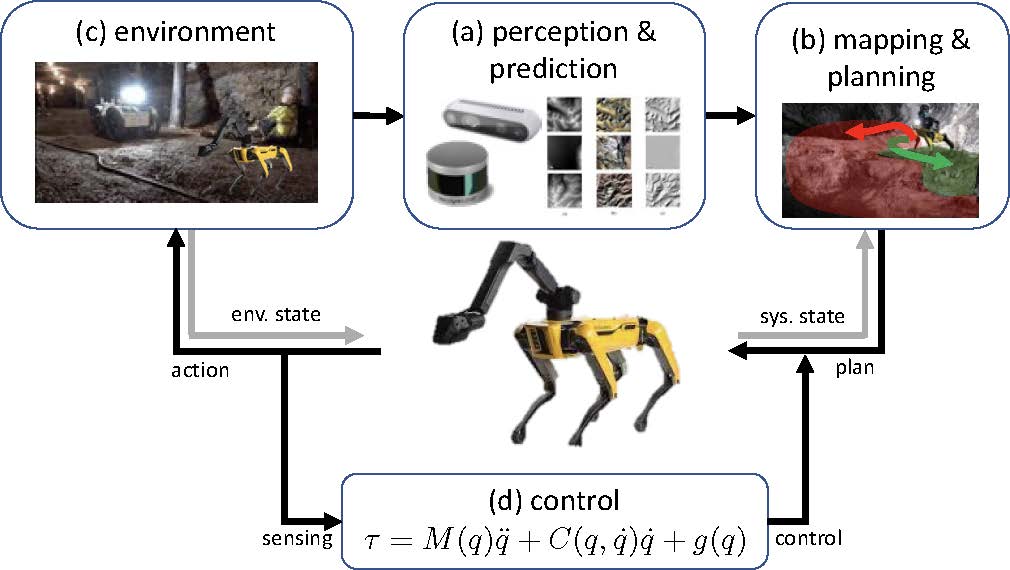
Dr. Matni and Dr. Pappas plan to test their work with a quadrupedal robot in a search-and-rescue scenario in an environment with unpredictable terrain and weather conditions. However, the goal is for the methods developed for this project to be applicable to a wide range of learning-enabled systems. Dr. Matni also hopes to test the methods on quadrotors as well by utilizing the outdoor Vicon system at GRASP’s location on the Pennovation Campus in order to incorporate more factors of real world uncertainty into the online deployment phase.
While GRASP faculty members approached the NSF SLES call with different methods, they share the same excitement about the new possibilities that improved safety measures could bring to the field of artificial intelligence.
Dr. Matni believes that this could be an opportunity to make safety easier to employ in AI for lay people who are not trained on these methods. “This is a new program, and it’s a privilege and an honor to be part of the first batch of proposals to have been funded through this call. I think there’s clearly a need for these kinds of ideas and people just don’t know what to do. So there are versions of what we propose that are being done ad hoc all over the place,” he stated, “I think there’s a really important opportunity here to formalize this process and systematize it and make it something that you don’t have to be creative or clever to do, you can just kind of push a button and make it happen.”
Featured People
Assistant Professor, ESE
Joseph Moore Professor & Department Chair, ESE