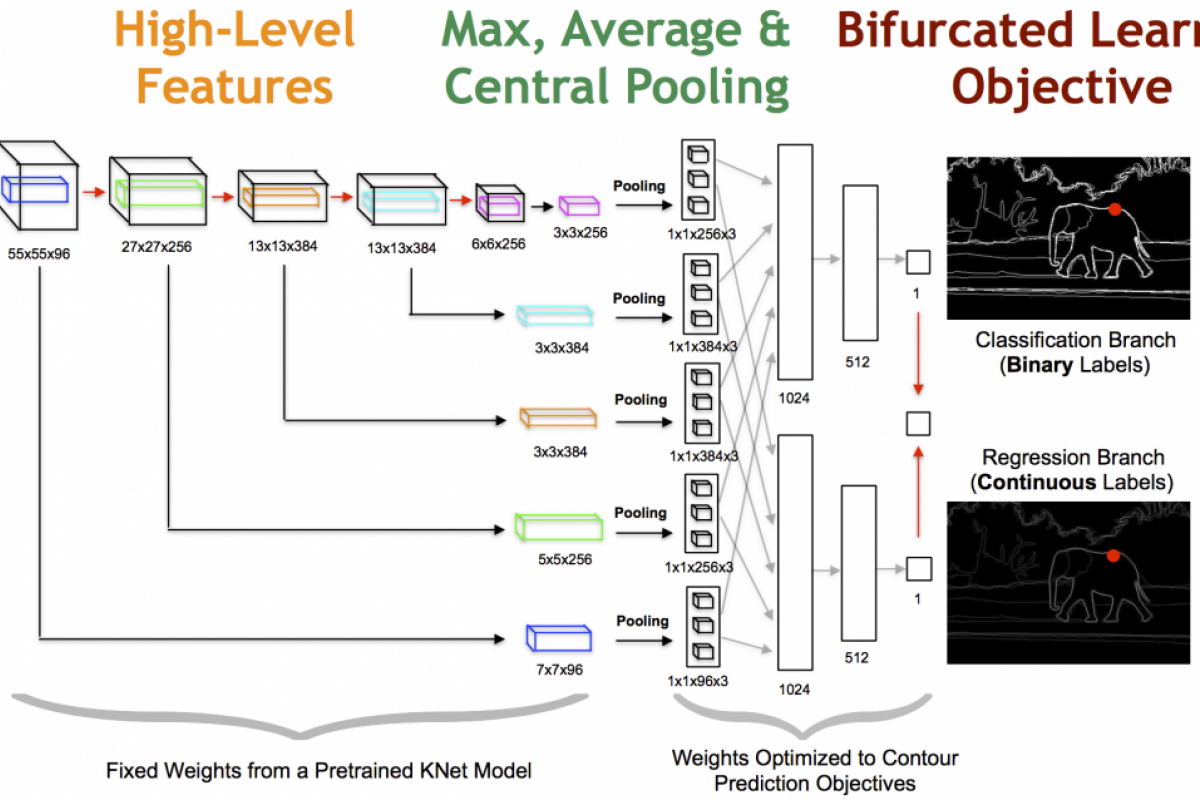
DeepEdge: A Multi-Scale Bifurcated Deep Network for Top-Down Contour Detection
Contour detection has been a fundamental component in many image segmentation and object detection systems. Most previous work utilizes low-level features such as texture or saliency to detect contours and then use them as cues for a higher-level task such as object detection. However, we claim that recognizing objects and predicting contours are two mutually related tasks. Contrary to traditional approaches, we show that we can invert the commonly established pipeline: instead of detecting contours with low-level cues for a higher level recognition task, we exploit object-related features as high-level cues for contour detection.
We achieve this goal by means of a multi-scale deep network that consists of five convolutional layers and a bifurcated fully-connected subnetwork. The section from the input layer to the fifth convolutional layer is fixed and directly lifted from a pre-trained network optimized over a large scale object classification task. This section of the network is applied to four different scales of the image input. These four parallel and identical streams are then attached to a bifurcated subnetwork consisting of two independently trained branches. One branch learns to predict the contour likelihood (with a classification objective) whereas the other branch is trained to learn the fraction of human labelers agreeing about the contour presence at a given point (with a regression criterion).
We show that without any feature engineering our multi-scale deep learning approach achieves state-of-the-art results in contour detection.
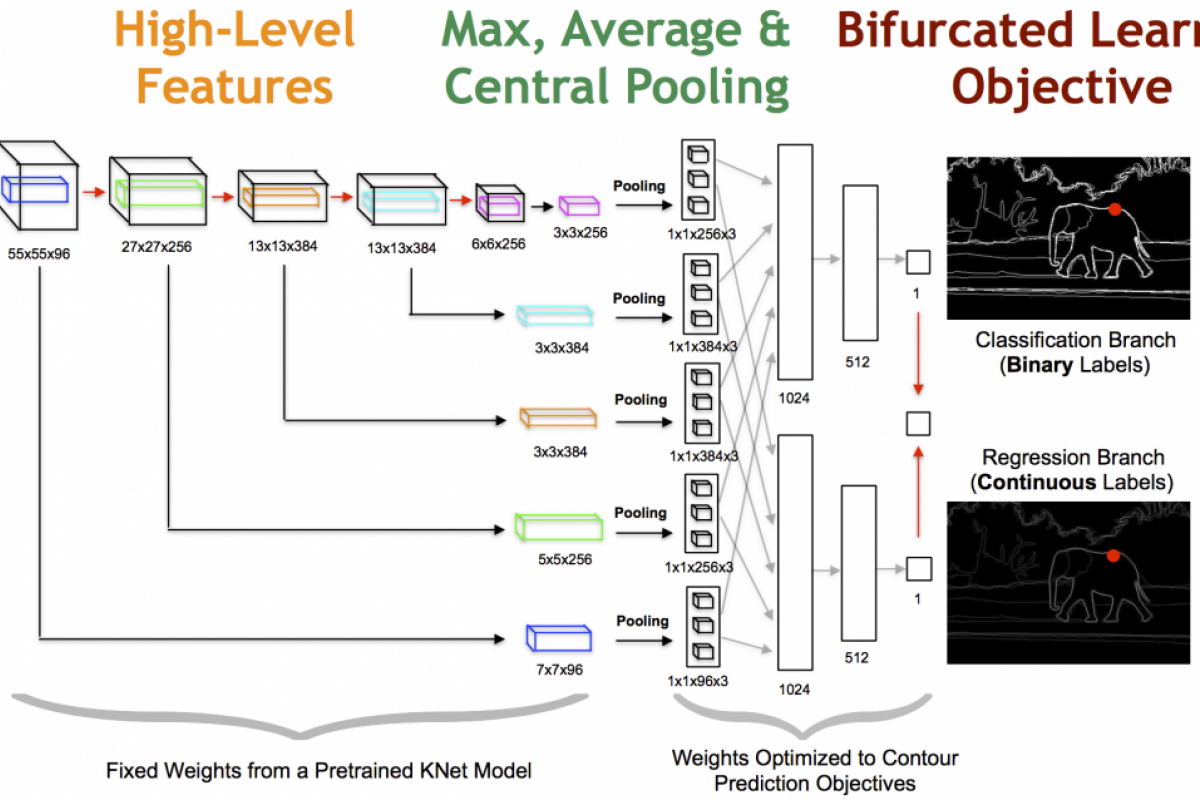